AI solutions for identifying fraud, waste, and abuse (FWA) in insurance claims
The values and opportunities
It is no secret that the healthcare system is complex. Fraud, waste and abuse (FWA) costs the industry hundreds of billions of dollars each year. A 2018 NHCAA report says, “some government and law enforcement agencies place the loss as high as 10% of our annual health outlay.” With the current expenditure over $4.1 trillion, the estimated annual cost of FWA in U.S. healthcare today is over $400 billion.
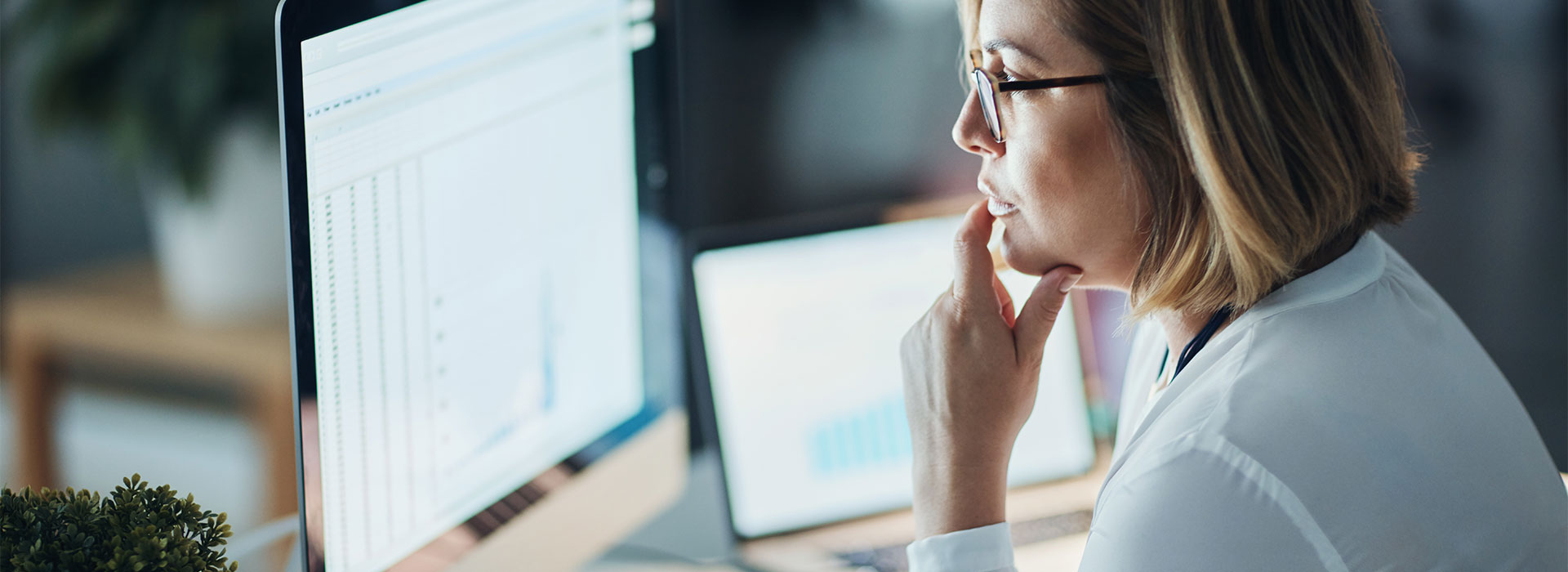
In addition to direct waste, the huge amount of FWA in the healthcare system eventually leads to higher health insurance fees and out-of-pocket expenses.
This is a huge loss every year but also a huge opportunity to create working solutions for fighting FWA and saving billions of dollars annually. Anti-FWA solutions can save 10-20% in healthcare expenditures by identifying fraudulent insurance claims. That is a large amount of money out of the pockets of fraudulent healthcare providers and into the pockets of patients and their insurance companies.
However, even when you do identify a candidate to launch an investigation on, you need to have some solid, data-driven reasons behind your choice because launching an investigation is not cheap. In fact, the average cost of opening and closing an investigation on a fraudulent healthcare case is $7,500 in the U.S.A.
AI solutions and the challenges
There are myriad methods of FWA in practice, but some of the more common examples are:
- Upcoding (provider submits for a more expensive diagnosis code)
- Unbundling (provider submits multiple codes for services with a single code)
- Drug diversion (when prescription medicines are obtained or used illegally)
- Billing for services not rendered
- Billing for more units than rendered
- Providing unnecessary services to members
- Alteration of records to get services covered
This list can go on and on with new patterns of FWA frequently being discovered and added. Criminals continually come up with new ideas to take advantage of the system through FWA patterns.
Identifying FWA patterns
Fighting FWA is an ongoing battle. Solutions must not only keep up to date about the known patterns of FWA but also keep trying to discover new unknown patterns.
Healthcare systems are complex, especially in the U.S., and AI-based anti-FWA solutions must deal with huge challenges. Some of these are regarding data such as data access, data structure, data cleaning, and so on. But the main challenge is the fact that labeled data is quite rare when it comes to FWA.
AI solutions for FWA come in two major categories depending on the availability of the FWA labels:
-
Supervised learning, where FWA labels are present (it is rare). In this case, developers can use supervised AI algorithms to train a model on the known patterns of FWA. The trained model then can be used to score any unseen data for the probability of FWA.
The best part is that the model evaluation is quite straightforward in a supervised learning approach. One can specifically measure the quality of the model based on comparing predictions and the ground truth. This approach works best for identifying the known patterns of FWA.
-
Unsupervised learning, where FWA labels are not present (most commonly). In this case, developers can use unsupervised AI algorithms to build a model that can still score the insurance claims on the probability of being different from a group of similar records.
The best part of the unsupervised learning approach is that one can discover new patterns of FWA that are not possible to detect by the supervised learning approach.
The main drawback of using unsupervised learning approaches is that the evaluation of the model can get quite challenging. The false alarm rate could get quite high because there is no ground truth (label) that can be used for model evaluation.
How to choose an anti-FWA solution
FWA is a huge space and there is a ton of work that needs to be done in terms of identifying and preventing FWA in scale, rapidly and reliably before making the final insurance payments.
Artificial intelligence models can be tailored to identify healthcare claims fraud, prescription abuse, up-charges and many additional FWA challenges.
Using the pattern recognition tools we’ve discussed allows advanced AI to create an end‐to‐end suite of profiling and modeling capabilities that continuously adapt and improve results. This seamless combination of tools can deliver personalized decisions in milliseconds to payers, insurance companies, business leaders, or other entities. These models evolve at scale with your data, increase detection rates, and decrease operational costs and false positives. In our work at Brighterion, we set this as a gold standard over the capability of legacy AI and solutions that are solely focused on rules-based systems.
The gold standard, according to Gartner, is to use a pre‐pay system that can vet payments for fraud before paying the health claim. Not only would a pre‐pay model prevent paying fraudulent claims, but it would also save on costly administrative time spent investigating false positives and allow investigators to focus on actual fraud.
When choosing an anti-FWA solution, look for features that include:
-
Diverse Expertise: The FWA team should consist of both top data scientists and experienced healthcare fraud investigators who will understand both sides of the equation.
-
Proven Solutions: Ensure the provider has successfully tested their solution end-to-end in the market and can demonstrate outstanding results. Look for attributes such as cloud-based platforms that offer real-time deployment at scale.
-
Flexible Options: How quickly can they deploy a custom solution? Do they have an out-of-the-box solution that one can leverage immediately with the right data requirements that still returns the desired results?
-
Secure Data/IPs: Is your data safe and secure? Ask about the provider’s data governance and how they follow best practices to ensure the safety and security of your data and their IP.
Conclusion
Identifying fraud, waste, and abuse in the healthcare system can save patients and insurance companies hundreds of billions of dollars annually. AI and machine learning offer significant help in this regard by modeling the known FWA patterns (supervised learning) and discovering the unknown FWA patterns (unsupervised learning).
Learn more about Mastercard's AI solutions for FWA that can be deployed anywhere in the pre-payment or post-payment lifecycle. Download our ebook, Prevent and save: advanced AI for fraud, waste and abuse.
Saeed Mirshekari, PhD is Director, Data Scientist at Brighterion, a Mastercard Company. After receiving his PhD in Physics from Washington University in Saint Louis in 2013, Saeed was a Postdoctoral Research Fellow at the International Center for Theoretical Physics in São Paulo, Brazil, and a researcher for the LIGO Scientific Collaboration. LIGO contributors were awarded a Special Breakthrough Prize in 2016 and a Nobel Prize for Physics in 2017 for their research in gravitational waves. Saaed entered data science in 2015 and joined Brighterion in 2020.